TITILE: Decomposable Graphical Models: On Learning, Fusion and Revision
Decomposable Graphical Models are of high relevance for complex industrial applications. The Markov network approach is one of their most prominent representatives and an important tool to structure uncertain knowledge about high dimensional domains. But also relational and possibilistic decompositions turn out to be useful to make reasoning in such domains feasible. Compared to conditioning the decomposable model on given evidence, the learning of the structure of the model from data as well as the fusion of several decomposable models is much more complicated. The important belief change operation revision has been almost entirely disregarded in the past, although the problem of inconsistencies is of utmost relevance for real world applications. In this talk these problems are addressed by presenting a successful complex application in an automotive industry.
DATE: May 24th, 2016
Rudolf Kruse
is Professor at the Otto-von-Guericke University of Magdeburg
(Germany), where he is leading the Computational Intelligence Group.
His current research interests include data science and intelligent systems.
His group is successful in various industrial applications in
cooperation with companies such as Volkswagen, Daimler, SAP, and British
Telecom.
He obtained his Ph.D. and his Habilitation in Mathematics from the Technical
University of Braunschweig in 1980 and 1984 respectively. Following a stay
at the Fraunhofer Gesellschaft, he joined the Technical University of
Braunschweig as a professor of computer science in 1986. Since 1996 he is a
full professor at the Department of Computer Science of the
Otto-Von-Guericke University of Magdeburg in Germany.
Rudolf Kruse has coauthored 15 monographs and 25 books as well as more
Than 350 refereed technical papers in various scientific areas.
He is a Fellow of the International Fuzzy Systems Association (IFSA), Fellow of the European
Coordinating Committee for Artificial Intelligence (ECCAI) and Fellow of the
Institute of Electrical and Electronics Engineers (IEEE).
To the top
Bernadette BOUCHON-MEUNIER
TITILE: Fuzzy and cognitive approaches of similarities
Fuzzy logic provides interesting tools for data mining and decision making, mainly because of its ability to represent imperfect information, for instance by means of imprecise categories, measures of resemblance or aggregation methods. This ability is of crucial importance when databases are complex, large, and contain heterogeneous, imprecise, vague, uncertain, incomplete data.
We focus our study on the use of similarities which are key concepts for all attempts to construct human-like automated systems or assistants to human task solving since they are very natural in the human process of categorization underlying many natural capabilities such as language understanding, pattern recognition or decision-making. We base our discourse on cognitive approaches of similarities, stemming for instance from Tversky's and Rosch's seminal works, among others.
We point out several types of measures of comparison compatible with these cognitive foundations, including measures of similarity and dissimilarity. We show that they can be involved in many steps of the process of data mining, such as clustering, construction of prototypes, fuzzy querying, for instance.
We eventually illustrate our discourse by examples of similarities used in real-world problems.
DATE: May 24th, 2016
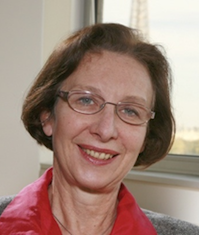
|
Bernadette Bouchon-Meunier
is a director of research emeritus at the National Centre for Scientific Research, the former head of the department of Databases and Machine Learning in the Computer Science Laboratory of the University Pierre et Marie Curie-Paris 6 (LIP6). She is the Editor-in-Chief of the International Journal of Uncertainty, Fuzziness and Knowledge-based Systems, the (co)-editor of 25 books, and the (co)-author of five. She has (co-)authored more than 400 papers on approximate and similarity-based reasoning, as well as the application of fuzzy logic and machine learning techniques to decision-making, data mining, risk forecasting, information retrieval, user modelling, sensorial and emotional information processing.
Co-executive director of the IPMU International Conference held every other year since 1986, she also served as the FUZZ-IEEE 2010 and FUZZ-IEEE 2013 Program Chair, the IEEE Symposium Series on Computational Intelligence (SSCI 2011) General Chair and the FUZZ-IEEE 2012 Conference Chair, as well as the Honorary chair of IEEE SSCI 2013 and IEEE CIVEMSA 2013.
She is currently the IEEE Computational Intelligence Society Vice-President for Conferences, the IEEE France Section Vice-President for Chapters and the IEEE France Section Computational Intelligence chapter chair. She is an IEEE fellow and an International Fuzzy Systems Association fellow. She received the IEEE Computational Intelligence Society Meritorious Service Award in 2012.
To the top
Jim Keller
TITLE: Recognition Technology: Lotfi’s look to the future from the late 1990s
In 1998, Lotfi Zadeh coined the term Recognition Technology, saying that it refers to current or future systems that have the potential to provide a "quantum jump in the capabilities of today’s recognition systems". Recognition Technology will include systems that incorporate three advances: new sensors, novel signal processing and soft computing. That vision has come to pass. I will discuss these three aspects of recognition technology through two quite different case studies that I am involved in: landmine detection and eldercare technology. They are both recognition systems. The former has a goal of detecting objects, explosive hazards, to help save lives while the latter focuses on recognizing human activities to allow older adults to live independently with a higher quality of life. While the sensors applied to these problems are dissimilar, they share many of the signal processing and pattern recognition approaches.
DATE: May 24th, 2016
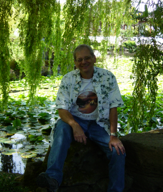
|
James M. Keller
received the Ph.D. in Mathematics in 1978. He holds the University of Missouri Curators’ Professorship in the Electrical and Computer Engineering and Computer Science Departments on the Columbia campus. He is also the R. L. Tatum Professor in the College of Engineering. His research interests center on computational intelligence: fuzzy set theory and fuzzy logic, neural networks, and evolutionary computation with a focus on problems in computer vision, pattern recognition, and information fusion including bioinformatics, spatial reasoning in robotics, geospatial intelligence, sensor and information analysis in technology for eldercare, and landmine detection. His industrial and government funding sources include the Electronics and Space Corporation, Union Electric, Geo-Centers, National Science Foundation, the Administration on Aging, The National Institutes of Health, NASA/JSC, the Air Force Office of Scientific Research, the Army Research Office, the Office of Naval Research, the National Geospatial Intelligence Agency, the Leonard Wood Institute, and the Army Night Vision and Electronic Sensors Directorate. Professor Keller has coauthored over 400 technical publications.
Jim is a Life Fellow of the Institute of Electrical and Electronics Engineers (IEEE) and the International Fuzzy Systems Association (IFSA), and a past President of the North American Fuzzy Information Processing Society (NAFIPS). He received the 2007 Fuzzy Systems Pioneer Award and the 2010 Meritorious Service Award from the IEEE Computational Intelligence Society. He finished a full six year term as Editor-in-Chief of the IEEE Transactions on Fuzzy Systems, followed by being the Vice President for Publications of the IEEE Computational Intelligence Society from 2005-2008, and has been an elected CIS Adcom member. He is the IEEE TAB Transactions Chair and a member of the IEEE Publication Review and Advisory Committee. Among many conference duties over the years, Jim was the general chair of the 1991 NAFIPS Workshop and the 2003 IEEE International Conference on Fuzzy Systems.
To the top
Christian Freksa
TITLE: From Fuzzy Sets to Spatial Cognition
Perception and cognition are resource-limited processes. They generate coarse information about environments that can be analyzed and described at arbitrary levels of resolution and abstraction. Fuzzy set theory and possibility theory provide a conceptual framework to characterize the relation between coarse information and high-precision ‘ground truth’ in environments and in mental states.
This relation – a mapping between coarse and fine entities – can be used in two ways: to infer high-precision information from coarse descriptions or to generate coarse descriptions from precise data. The mappings are particularly useful for interfacing high-resolution technical systems with human-friendly low-resolution systems such as natural language. In the interaction between cognitive agents such as humans and robots, we are confronted with a third mapping: the mapping between two coarse systems that do not have access to a common high-resolution ground truth.
From a theoretical perspective, such a coarse-to-coarse mapping can be viewed as a composition of a (fictitious) coarse-to-fine mapping composed with a fine-to-coarse mapping. However, the composition does not yield a useful model for the actual mechanisms that enable cognitive agents to successfully interact. In my talk I will describe how fuzzy sets and possibility theory serve as a framework for concepts in spatial cognition that permit natural interaction between cognitive agents in spatial environments.
DATE: May 24th, 2016
Christian Freksa
is Professor of Cognitive Systems at the Faculty of Mathematics and Informatics at the University of Bremen, Germany. He directs the Bremen Spatial Cognition Center. His research concerns representation and reasoning with incomplete, imprecise, lean, coarse, approximate, fuzzy, and conflicting knowledge about physical environments. Particular emphasis is on qualitative spatial and temporal reasoning. Freksa received a PhD in Artificial Intelligence from UC Berkeley. He carried out research at the Max Planck Institute and at the Technical University of Munich, at the International Computer Science Institute in Berkeley, and at the University of Hamburg. From 1996 to 2014 he directed national research initiatives on Spatial Cognition supported by the German Research Foundation (DFG). Freksa is a Fellow of the European AI society ECCAI.
To the top
Janusz Kacprzyk
TITLE: Fuzzy preferences and majority in multiagent decision making: from status quo to innovative decisions
Our main concern is the process of multiagent decision making under fuzzy preferences and fuzzy majority. We have a set of options and a set of agents, human beings or not, who present their testimonies assumed to be fuzzy preference relations. We look for an option (or a set of options) which is best acceptable by the group of agents, i.e. by a fuzzy majority (e.g. most) of them. We deal with relatively small sets of options and agents. The fuzzy majority, introduced by Kacprzyk (1985), is represented by a fuzzy linguistic quantifier like “most”, “almost all”, “much more than a half”, etc. dealt with by, e.g., Zadeh’s calculus of linguistically quantified propositions or Yager’s OWA operators. Then, from the fuzzy preferences of the individual agents we determine some solution concept like Kacprzyk’s fuzzy Q-core, i.e. a fuzzy set of options which are not defeated in pairwise comparisons by the required fuzzy majority Q (e.g. most) of agents. This solution concept can then be extended by taking into account the importance of agents and relevance of options. First, we follow the usual path in this area, i.e. we claim that a “better” group decision can be found if agents are at consensus. We perform a consensus reaching session, find consensual fuzzy preferences, and determine a fuzzy set of agent whose fuzzy preferences are close to the consensual ones; this implies what we call status quo solutions. Then, taking another point if departure, related to some claims of knowledge and innovation management, we find another fuzzy set of agents whose preferences are far from the consensual ones; this implies what we call innovative solutions. We show, as a possible way to obtain those status quo and innovative solutions, new augmented fuzzy Q-cores for those consensory and dissensory agents, and analyze the solutions obtained from the point of view of their novelty and innovativeness which are measured by using some numerical indexes. We show some example of choosing a regional development policy.
DATE: May 25th, 2016
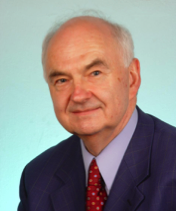
|
Janusz Kacprzyk
graduated from Warsaw University of Technology, Poland, with M.Sc. in automatic control and computer science, obtained in 1977 Ph.D. in systems analysis and in 1991 D.Sc. in computer science. He is Professor of Computer Science at the Systems Research Institute, Polish Academy of Sciences, and at WIT – Warsaw School of Information Technology, and Professor of Automatic Control at PIAP – Industrial Institute of Automation and Measurements, and Department of Electrical and Computer Engineering, Cracow University of Technology. He is Honorary Foreign Professor at the Department of Mathematics, Yli Normal University, Xinjiang, China, and Visiting Scientist at RIKEN Brain Research Institute, Tokyo, Japan. He is Full Member of the Polish Academy of Sciences, Member of Academia Eueopaea (Informatics), Member of European Academy of Sciences and Arts (Technical Sciences), Foreign Member of the Spanish Royal Academy of Economic and Financial Sciences (RACEF), and Foreign Member of the Bulgarian Academy of Sciences. He is Fellow of IEEE, IFSA, ECCAI and MICAI.
He has been a frequent visiting professor in the USA, Italy, UK, Mexico, China. His main research interests include the use of modern computation computational and artificial intelligence tools, notably fuzzy logic, in decisions, optimization, control, data analysis and data mining, with applications in databases, ICT, mobile robotics, etc.
He authored 5 books, (co)edited more than 80 volumes, (co)authored ca. 500 papers. His bibliographic data are: due to Google Scholar - citations: 19765; h-index: 64, due to Scopus: citations: 4606; h-index: 32; due to WoS: citation: 3821, h-index: 27. He is the editor in chief of 6 book series at Springer, and of 2 journals, and is on the editorial boards of ca. 40 journals. He is a member of the Adcom of IEEE CIS, and was a Distinguished Lecturer of IEEE CIS.
He received many awards: 2006 IEEE CIS Pioneer Award in Fuzzy Systems, 2006 Sixth Kaufmann Prize and Gold Medal for pioneering works on soft computing in economics and management, 2007 Pioneer Award of the Silicon Valley Section of IEEE CIS for contribution in granular computing and computing in words, 2010 Award of the Polish Neural Network Society for exceptional contributions to the Polish computational intelligence community, IFSA 2013 Award for his lifetime achievements in fuzzy systems and service to the fuzzy community, and the 2014 World Automation Congress Lifetime Award for contributions in soft computing. He is President of the Polish Operational and Systems Research Society and Past President of International Fuzzy Systems Association.
To the top
Vladik Kreinovich
TITLE: Fuzzy Logic Can Justify and Improve Semi-Heuristic Data and Image
Processing Techniques: Main Idea and Case Studies
Fuzzy logic techniques were originally designed to translate
expert knowledge -- which is often formulated by using imprecise
("fuzzy") from natural language (like "small") -- into precise
computer-understandable models and control strategies. Such a
translation is still the main use of fuzzy techniques. For
example, we want to control a complex plant for which no good
control technique is known, but for which there are experts how
can control this plant reasonably well. So, we elicit rules from
the experts, and then we use fuzzy techniques to translate these
rules into a control strategy.
Lately, it turned out that fuzzy techniques can help in another
class of applied problems: namely, in situations when there are
semi-heuristic techniques for solving the corresponding problems,
i.e., techniques for which there is no convincing theoretical
justification. Because of the lack of a theoretical justification,
users are reluctant to use these techniques, since their previous
empirical success does not guarantee that these techniques will
work well on new problems.
Also, these techniques are usually not perfect, and without an
underlying theory, it is not clear how to improve their
performance. For example, linear models can be viewed as first
approximation to Taylor series, so a natural next approximation is
to use quadratic models. However, e.g., for l^p-models, when they
do not work well, it is not immediately clear what is a reasonable
next approximation.
In this talk, we show that in many such situations, the desired
theoretical justification can be obtained if, in addition to known
(crisp) requirements on the desired solution, we also take into
account requirements formulated by experts in natural-language
terms. Naturally, we use fuzzy techniques to translate these
imprecise requirements into precise terms. To make the resulting
justification convincing, we need to make sure that this
justification works not only for one specific choice of fuzzy
techniques (i.e., membership function, "and"- and "or"-operations,
etc.), but for all combinations of such techniques which are
consistent with the corresponding practical problem.
As examples, we provide the detailed justification of:
1) sparsity techniques in data and image processing -- a very
successful hot-topic technique whose success is often largely a
mystery;
2) l^p-regularization techniques in solving inverse problems --
an empirically successful alternative to Tikhonov regularization
appropriate for situations when the desired signal or image is not
smooth; and
3) non-linear empirical models of soil mechanics used in road
construction.
Several other applications will be mentioned.
DATE: May 25th, 2016
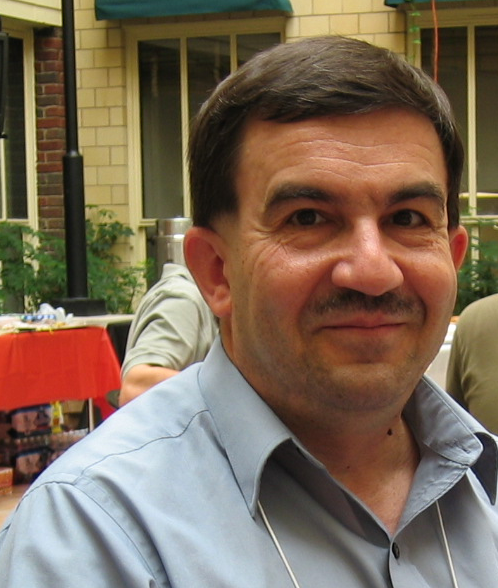
|
Vladik Kreinovich
received his MS in Mathematics and Computer
Science from St. Petersburg University, Russia, in 1974, and PhD
from the Institute of Mathematics, Soviet Academy of Sciences,
Novosibirsk, in 1979. From 1975 to 1980, he worked with the Soviet
Academy of Sciences; during this time, he worked with the Special
Astrophysical Observatory (focusing on the representation and
processing of uncertainty in radioastronomy). For most of the
1980s, he worked on error estimation and intelligent information
processing for the National Institute for Electrical Measuring
Instruments, Russia. In 1989, he was a visiting scholar at Stanford
University. Since 1990, he has worked in the Department of Computer
Science at the University of Texas at El Paso. In addition, he has
served as an invited professor in Paris (University of Paris VI),
France; Hong Kong; St. Petersburg, Russia; and Brazil.
His main interests are the representation and processing of
uncertainty, especially interval computations and intelligent
control. He has published six books, eleven edited books, and
more than 1,100 papers. Vladik is a member of the editorial board
of the international journal "Reliable Computing" (formerly
"Interval Computations") and several other journals. In addition,
he is the co-maintainer of the international Web site on interval
computations
http://www.cs.utep.edu/interval-comp .
Vladik is Vice President for Publications of IEEE Systems, Man,
and Cybernetics Society; he served as President of the North
American Fuzzy Information Processing Society 2012-14; is a
foreign member of the Russian Academy of Metrological Sciences;
was the recipient of the 2003 El Paso Energy Foundation Faculty
Achievement Award for Research awarded by the University of Texas
at El Paso; and was a co-recipient of the 2005 Star Award from the
University of Texas System.
To the top
Ildar Batyrshin
TITLE: Fuzzy Logic and Non-Statistical Association Measures
The idea of measuring of positive and negative associations (or correlations) between data is very popular in data analysis. The Pearson’s correlation coefficient as a measure of association is used in many approaches to data analysis, machine learning, signal processing etc. This correlation coefficient serves as a prototype for construction of association measures in different application areas.
This talk will discuss the general methods of construction of association measures as functions satisfying the properties of Pearson’s correlation coefficient. These methods use the technique developed in the theory of fuzzy logic and aggregation functions. Examples of association and correlation measures constructed by these methods on different domains are discussed. The measures of associations and correlations on [0,1], on the set of fuzzy set, on the set of time series etc. are considered.
DATE: May 25th, 2016
Ildar Batyrshin
graduated from the Moscow Physical-Technical Institute, Faculty of Control and Applied Mathematics in 1975. He received his PhD from the Moscow Power Engineering Institute in 1983 and Dr. Sc. (habilitation) from the Higher Attestation Committee of Russian Federation in 1996. He joined the Department of Informatics and Applied Mathematics of Kazan State Technological University, Kazan, Russia in 1975, and served as the Department Head in 1997-2003. Since 1999, he was also a leading researcher of the Institute of Problems of Informatics of Academy of Sciences of the Republic of Tatarstan, Russia. He joined the Research Program of Applied Mathematics and Computations of Mexican Petroleum Institute in 2003 as a leading researcher. Since 2014 he is with the Center for Computing Research of Mexican National Polytechnic Institute (CIC IPN) as a Titular Professor C.
He is a Past President of Russian Association for Fuzzy Systems and Soft Computing (RAFSSoftCom), a member of the Council of Mexican Society for Artificial Intelligence, a member of the Board of Directors of NAFIPS, Senior Member of IEEE Computational Intelligence Society, a member of editorial boards of several scientific journals.
His awards: Research Level 2 of the National System of Researchers of Mexico; Best Research Prize of Mexican Petroleum Institute in 2007 for the Development of Fuzzy Expert System in Water Production Diagnosis; Honorary Professor of Obudu University, Budapest, Hungary; Honorary Researcher of the Republic of Tatarstan, Russia; State Research Fellowship of the Presidium of Russian Academy of Sciences for Distinguished Researchers (1997-2003).
He served as a Co-Chair of 9 International Conferences on Soft Computing, Artificial Intelligence and Computational Intelligence. He is a co-author and co-editor of 19 books and special volumes of journals. He is an author of more than 200 scientific publications.
To the top
Michio Sugeno
TITLE: Explorations in Ordinal Preference Theory with the Savage’s Omelet Problem
There are two preference models used in preference theory for multi-criteria decision making: cardinal models with addition-multiplication on real numbers and ordinal models with max-min operations on ordinal numbers.
In this study, we explore ordinal preference models based on various S-integrals which are counterparts of various Choquet integrals used as cardinal preference models. We examine a family of S-integrals such as S-integrals, Symmetric S-integrals, Cumulative Prospect Theory S-integrals, Bi-Capacity S-integrals and Level-Dependent S-integrals through the Savage’s Omelet Problem which has been often used as a simple illustrative example in multi-criteria decision making. In this problem, however, we find many preference orders of acts depending on the consequents of acts. In the examinations, we find that there exist some preference orders which cannot be modeled even by Bi-Capacity S-integrals and Level-Dependent S-integrals. In order to completely solve the problem, we successfully apply a novel modeling scheme called Hierarchical S-integrals.
Finally, reviewing the history of cardinal preference models, we introduce an essential concept of ‘admissibility’ into preference theory. With this concept, we can clearly state the primary objective of preference theory. Considering it, we pose a hypothesis that any admissible preference orders could be modeled with our Hierarchical S-integrals in the case of ordinal preference or Hierarchical Choquet integrals in the case of cardinal preference.
DATE: May 25th, 2016
Michio Sugeno
was born in Yokohama; Japan in 1940. After graduating from the Department of Physics, the University of Tokyo, he worked at a company for three years. Then, he served the Tokyo Institute of Technology as Research Associate, Associate Professor and Professor from 1965 to 2000. After retiring from the Tokyo Institute of Technology, he worked as Laboratory Head at the Brain Science Institute, RIKEN from 2000 to 2005, as Distinguished Visiting Professor at Doshisha University from 2005 to 2010 and then as Emeritus Researcher at the European Centre for Soft Computing, Spain from 2010 to 2015. He is Emeritus Professor at the Tokyo Institute of Technology.
He was President of the Japan Society for Fuzzy Theory and Systems from 1991 to 1993, and also President of the International Fuzzy Systems Association from 1997 to 1999. He is the first recipient of the IEEE Pioneer Award in Fuzzy Systems with Zadeh in 2000. He also received the 2010 IEEE Frank Rosenblatt Award and Kampét de Feriét Award in 2012.
His research interests are Choquet calculus, fuzzy measure theory, nonlinear control, preference theory, applications of Systemic Functional Linguistics and language functions of the brain.
To the top