Special Session Chairs
Bernadette Bouchon-Meunier
University of Paris 6, France
Davood Rafiei
University of Alberta, Canada
Richard De Veaux
Williams College, USA
Special sessions play a big role in promoting relevant focussed areas that are
not well covered in the main conference tracks, bringing together researchers,
industry experts, practitioners and potential users who are interested in different aspects of data science and analytics.
DSAA'2016 features 8 special sessions as listed here. Both the paper submission date and the notification date for all special sessions are the same as those of the main conference tracks. When you submit a paper to a special session, select the respective special session in the IEEE DSAA'2016 submission page. Review will be coordinated by the special session chairs and final decisions will be made by the program co-chairs. Accepted special session submissions will be included into the Special Session part of the main conference proceedings to be published by IEEE and included into the IEEE Xplore Digital Library.
- Special Session on Big Behavioral Data Analytics (BBDA)
- Special Session on Data Science for Agricultural Decision Support Systems (DS4ADSS)
- Special Session on Environmental and Geo-spatial Data Analytics (EnGeoData)
- Special Session on Game Data Science (GDS)
- Special Session on Health Data Science (HDS)
- Special Session on Emotion and Sentiment in Intelligent Systems and Big Social Data Analysis (SentISData)
- Special Session on Statistical and Mathematical Tools for Data Mining (SMTDM)
- Special Session on Statistical Learning for Data Science (SLDS)
- Special Session on Integration of Statistics and Data Science (ISDS)
Special Session on Big Behavioral Data Analytics (BBDA)
Chairs:
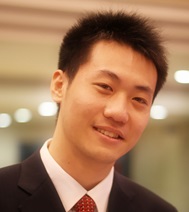 |
|
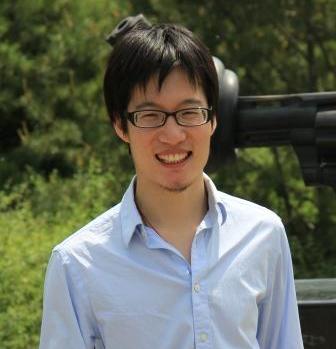 |
|
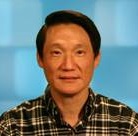 |
|
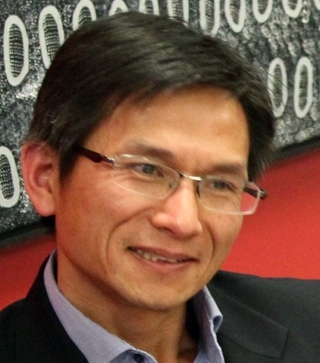 |
Meng Jiang |
|
Peng Cui |
|
Philip S. Yu |
|
Longbing Cao |
University of Illinois at Urbana-Champaign, USA |
|
Tsinghua University, China |
|
University of Illinois at Chicago, USA |
|
University of Technology Sydney, Australia |
Aims and Scope
With the rapid proliferation of web applications, such as search engine, e-commerce and social networking service, more and more user behaviors are available online, which opens a new perspective for behavioral data analytics where more focus should be put on various types of interactions on the web. For example, users can build friendships with, send messages to and make phone calls with other users, creating user-user interactions; they can also post messages, buy products and check in restaurants, creating user-item interactions. Developing computational methods to model user behaviors, analyze different behavioral patterns, understand mechanisms underlying behavioral logs and eventually predict the next behaviors or detect strange behaviors is of paramount importance since it would improve applications like web search, recommender system and social networking services and, on the other side, stop frauds, spams and attacks. This presents clear challenges to behavior modeling: user behavior depends on contents, intentions and contexts in complex online environments. Moreover, the online settings bring big challenges to behavioral data analysis since user behavioral data is in web scale, heterogeneous, of multiple dimensions, highly sparse and dynamic.
Topics of Interest:
Methods and techniques:
- New principles of user behavior formation
- Modeling personal preference and interpersonal influence
- Modeling individual behavior and group behavior
- Modeling temporal behavior and behavioral dynamics
- Modeling check-in behavior and purchasing behavior
- Scalable techniques for large-scale behavioral data analysis
- Efficient techniques for online behavioral processing
Applications of online user behavioral analytics, such as:
- Social networks
- Recommender systems
- E-commerce systems
- Fraud and spam detection
- Suspicious behavior detection
- Search engines
Special Session on Data Science for Agricultural Decision Support Systems (DS4ADSS)
URL:
https://www.iiit.ac.in/DS4ADSS/
Chairs:
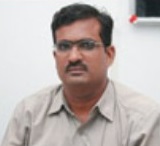 |
|
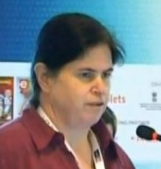 |
Krishna Reddy |
|
Leisa Armstrong |
IIITH, Gachibowli, Hyderabad, India |
|
Edith Cowan University, Australia |
Aims and Scope
With increasing world population, there is a need to improve agricultural productivity in sustainable manner. Great efforts are being made by researchers to exploit the potential of ICTs and develop ICT-driven systems to help farmers and stakeholders make better decisions related to crop and livestock management strategies. The special session focuses on data science based approaches to build next generation agricultural decision support systems to help farmers and other stakeholders.
Topics of Interest:
Innovative methodologies and frameworks for building agricultural decision support systems based on the data science related to:
- crop production,
- agriculture education and extension,
- soil management,
- water management,
- weather-based crop management,
- agri business and marketing, and
- livestock and fisheries.
The corresponding data science approaches are in, but not limited to, the following areas:
Data processing,
Image processing,
Visual information processing,
Speech processing,
Data aggregation,
Data summarization,
Semi-structured data management,
Distributed systems,
Information extraction,
Information retrieval,
Information integration,
Information dissemination,
Information visualization,
Content development and organization,
Crowd sourcing,
Big data analytics,
Sensor data processing,
Data mining,
Artificial intelligence,
Machine learning,
Internet of things,
Geospatial techniques,
Social networks,
Cloud computing,
Pattern recognition,
Multiagent systems, and
Game theory.
Special Session on Environmental and Geo-spatial Data Analytics (EnGeoData)
Chairs:
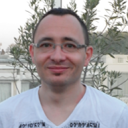 |
|
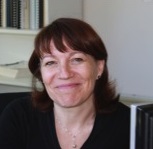 |
|
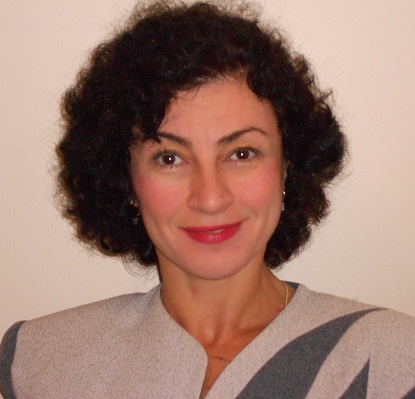 |
Mathieu Roche |
|
Maguelonne Teisseire |
|
Diana Inkpen |
Cirad, TETIS & LIRMM, France |
|
Irstea, TETIS & LIRMM, France |
|
Unversity of Ottawa, Canada |
Aims and Scope
Environmental and more generally geo-spatial information is now provided by crowdsourcing but also by public administrations in the context of the open data policies. Analyses of such data are still challenging. Firstly because of their heterogeneity (structural, semantic, spatial and temporal), and secondly because of the difficulty in choosing the "best" knowledge discovery process to apply, according to the needs of the experts in the field. This special issue aims to provide high quality research covering all or part of the challenges mentioned above, from a theoretical or experimental point of view.
Challenge about data science deals with creation, storage, search, sharing, modeling, analysis, and visualization of data, information, and knowledge. In Data Science context, spatio-temporal aspects are crucial in order to manage and mine data, to index and retrieve information, and finally to discover and visualize knowledge. By taking into account these spatio-temporal aspects, original methods have to be proposed for processing real and complex data from different domains, e.g., environment, agriculture, health, urban, and so forth.
Topics of Interest:
- Pre and Post Data processing
- Data Quality, and Result Evaluation
- Data Mining or Data Warehousing Applications
- Text-Mining or Information Retrieval
- Visual Analytics
- KDD real use-cases dedicated to environmental and geo-spatial Data
Special Session on Game Data Science (GDS)
URL:
http://gamedatascience.org
Chairs:
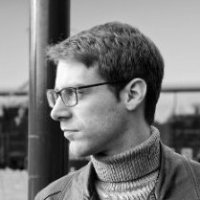 |
|
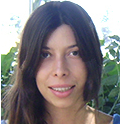 |
Alain Saas |
|
Africa Perianez |
Silicon Studio, Japan |
|
Silicon Studio, Japan |
Aims and Scope
In the past years, both traditional video game platforms and emerging mobile games have tended to become always-connected to the internet. This allows game developers to collect a huge amount of data in real time while maintaining an active relationship with the players.
This evolution also contributed to the reach of a wider audience for video games (casual gamers) and to the appearance of new economic models (in-app-purchases, Free-To-Play) that are taking more and more importance in a sector traditionally monetized by expensive one-time purchases or sometimes subscriptions.
This recent revolution of the video-game industry creates a broad range of new challenges for both research and business applications. The interest of the industry is reflected by the quickly growing number of job openings for data scientists in game companies.
The current trend to include social features and in-app-purchases to games, combined with the exceptional level of granularity of the data collected makes game datasets a unique source of information to observe and analyze human behavior, including social and consumer dynamics. Therefore, it can be anticipated that the outcomes of game data science research will benefit other sectors e.g. other emerging mobile data-driven applications, online marketing, retail, e-commerce.
It is paramount that research efforts focus on the development of adequate statistical and learning methods able to model and predict player behavior, that scale to big datasets and allows an intuitive visualization of the results.
The special session on Game Data Science aims to bring together experts from research and industry, and to provide a stimulating atmosphere to promote collaborations and mutual exchange. The goal of the GDS session is to gather outstanding contributions, pursuing the development and application of new technologies towards a new paradigm in video-games.
Topics of Interest:
Machine learning applied to game datasets
- Advanced methods
- Dimensionality reduction and feature extraction
- Modeling of the player behavior and social interactions
- Churn prediction
- Forecast of time series
- Forecast of the impact of game and marketing events on player behavior
- Clustering of player profiles and activity
- Virality models
Deployment of game data science in products
- Big data architecture challenges
- Novel algorithms that scale with big datasets
- A/B testing of game data science features
- Visualizations and visual analytics
- Novel visualization techniques for time-series analysis
- Game data science product management
- Game data science applied to game development
Special Session on Health Data Science (HDS)
Chairs:
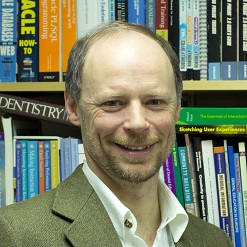 |
|
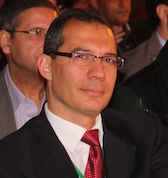 |
|
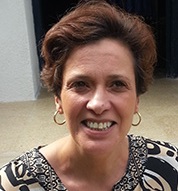 |
|
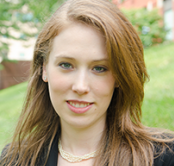 |
Titus K. Schleyer |
|
Nabil Abdennadher |
|
Zina Ben Miled |
|
Sherri Rose |
Regenstrief Institute Inc., Indiana University, USA |
|
University of Applied Sciences, Western Switzerland |
|
Indiana University Purdue University - Indianapolis, USA |
|
Harvard University, USA |
Aims and Scope
The health sector has been recently experiencing an increasing accessibility and availability of public and private data from various sources. This wide range of data sources are the result of 1) the continuing investment in the digitization of health records, 2) the availability of an increasing number of health-related mobile and web-enabled applications, and 3) the use of social media for community-focused health research. This data presents a unique and cost-effective opportunity for knowledge discovery and has the potential to accelerate research while enabling the translation of the research findings to direct benefits to the community.
This session brings together scientists, engineers, and researchers from academia and industry in order to discuss
-
The development of algorithms, tools and techniques that can enhance our understanding of health data;
- The use of large data sets to conduct health-focused studies; and
- The use of social networks to influence community behavior.
Contributions that clearly demonstrate the benefits of large scale studies and systems as opposed to traditional studies and systems are highly solicited.
Topics of Interest:
Case studies that extract knowledge from social media, web databases, electronic health records,accelerometers or large data warehouses including but not limited to emotional behavior, epidemiology, health economics, genomics, sentiment analysis and trend analysis;
Development of algorithms that enhance our understanding of health data;
Software applications that are used to collect, manage and disseminate large scale health-related information;
High performance computing for health applications;
Mechanisms and approaches for evaluating and validating findings from large scale social networks;
Novel methodologies and applications that combine traditional and emerging methods to accelerate research;
Applications that answer important scientific questions with implications for health policy; and
Case studies highlighting the use of social networks for preventive medicine or enhancing health awareness in the community
Special Session on Emotion and Sentiment in Intelligent Systems and Big Social Data Analysis (SentISData)
Chairs:
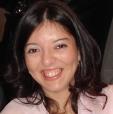 |
|
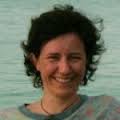 |
|
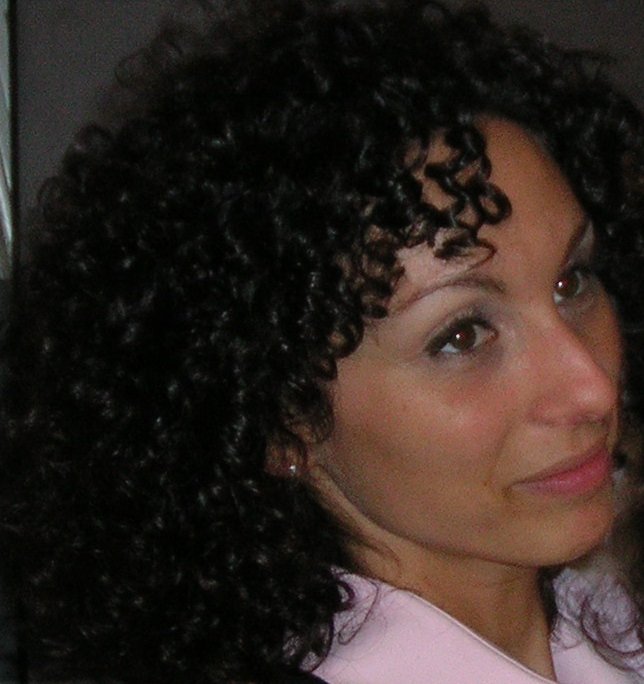 |
|
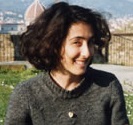 |
|
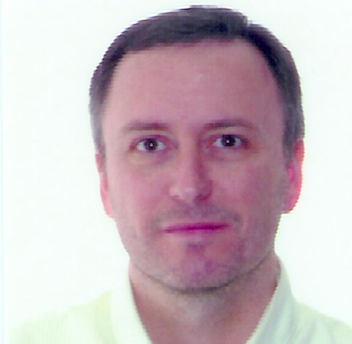 |
Farah Benamara Zitoune |
|
Cristina Bosco |
|
Elisabetta Fersini |
|
Viviana Patti |
|
Emilio Vivancos |
University of Toulouse, France |
|
University of Torino, Italy |
|
University of Milano-Bicocca, Italy |
|
University of Torino, Italy |
|
Polytechnic University of Valencia, Spain |
Aims and Scope
The rise of social media and the availability of big social data represent a challenge and a push forward for research on emotion and sentiment, which can meaningfully contribute to the investigation on affective cognitive models and their integration into intelligent systems.
Social media are typical contexts for the emergence of subjective and expressive dimensions, but both the huge amount of data available and the relative dilution, within these data, of the meaning to be extracted, have to be carefully taken into account. Moreover, the role of the affective dimension is crucial also for systems interacting with humans in communicating data, where affect can contribute to convey the complex meanings underlying data.
This calls for delving into the evolution of approaches, techniques and tools for modeling and analyzing emotion and sentiment, with the aim of dealing with the affective information conveyed by media that reflect spontaneous, unstructured user responses, and applying big social data analysis within a dynamic corpus of contents, created and enriched by users according to new paradigms of interactions fostering emotional engagement.
Big social data analysis is interdisciplinary and combines areas such as natural language processing, social network analysis, multimedia management, social media analytics, trend discovery, information retrieval, computational linguistics. The goal of this special session is to collect contributions on the development and application of techniques for analyzing big social data, with a special focus on sentiment analysis and opinion mining, and on research about paradigms for the integration of emotional states in intelligent systems, to improve systems both for what concerns emotion-awareness and affective human-computer interaction.
Topics of Interest:
- Emotion and sentiment in social media and big data
- Subjectivity, sentiment and emotion detection in social media texts
- Sentiment-based indexing, search and retrieval in social media
- Sentiment topic detection & trend discovery
- Irony and sarcasm detection
- Multimodal emotion and sentiment detection in social media
- Emotion, sentiment and social network analysis
- Time evolving opinion & sentiment analysis- Emotions, sentiment, geographic locations and places
- Using big social data to measure emotional states (e.g. happiness, anger, disappointment)
- Using sentiment and affect for social media predictive analysis including genre identification, political preference, etc.
- Emotion models and ontologies of emotions
- Affect in natural language
- Emotion-aware intelligent systems
Special Session on Statistical and Mathematical Tools for Data Mining (SMTDM)
Chairs:
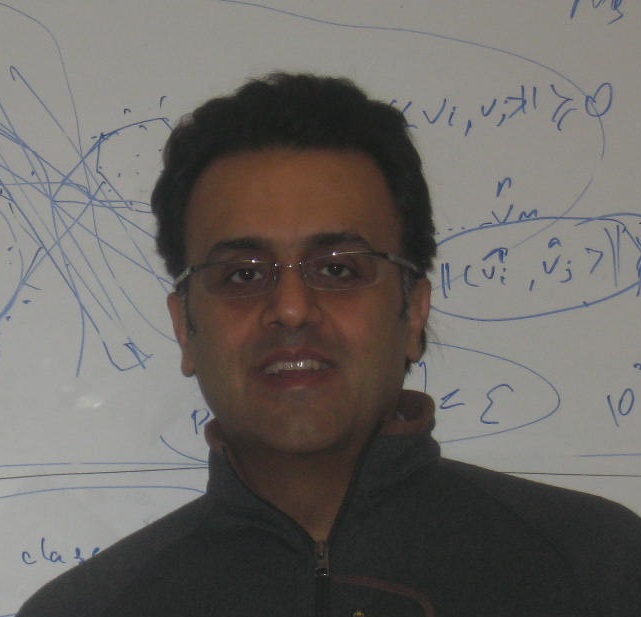 |
|
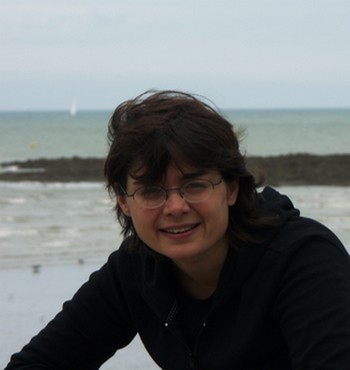 |
|
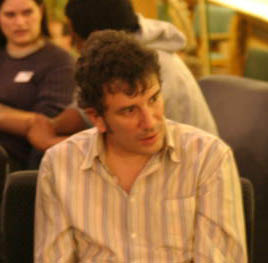 |
Massih-Reza Amini |
|
Marianne Clausel |
|
Eric Gaussier |
University of Grenoble, France |
|
University of Grenoble, France |
|
University of Grenoble, France |
Aims and Scope
Huge amounts of data are now easily and legally available on the Web.
This data is generally heterogeneous and merely structured.
Data mining and Machine learning models which have been developed to automatically retrieve,
classify or cluster observations on large yet homogeneous data collections have to be rethought.
Indeed, many challenging problems, inevitably associated to Big Data, have manifested the needs for tradeoffs between the two conflicting goals of speed and accuracy.
This has led to some recent initiatives in both theory and practice from different communities as machine learning, data mining and statsitics.
The goal of this special session is to bring together research studies aiming at developing new data mining and machine learning tools to handle new challenges associated to data science.
Topics of Interest:
- Distributed on-line learning
- Multi-task learning for big data
- Transfer Learning for big data
- Optimization techniques for large-scale learning
- Handling large number of target classes in big data
- Structured prediction models in big data
- Speed/Accuracy tradeoffs in big data
- Statistical inference for big data
- Noise in Big data
Special Session on Statistical Learning for Data Science (SLDS)
Chairs:
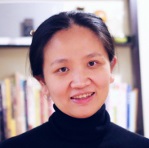 |
|
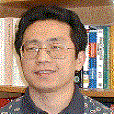 |
|
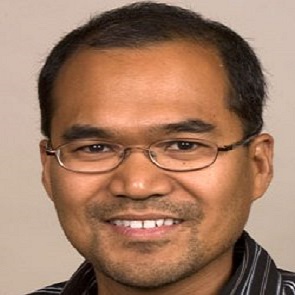 |
Tian Zheng |
|
Wei Pan |
|
Hernando Ombao |
Columbia University, USA |
|
University of Minnesota, USA |
|
University of California at Irvine, USA |
Aims and Scope
Statistics plays a central role in the data science approach. This
special session is to engage statisticians who study methods and theory that are
fundamental to data science. Recent advances in statistical learning and modeling for
complex data will be presented. Extended versions of accepted papers to this special
session will be considered for a special issue of Statistical Analysis and Data
Mining, the ASA data science journal.
Topics of Interest:
- Advances in theory or models associated with the analysis of massive, complex
datasets
- Statistical modeling and data mining for data-driven solutions of real-world
problems
- Innovative data mining algorithms or novel statistical approaches
- Compare and contrast techniques to solve a problem, along with an objective
evaluation of the analyses and the solutions
Special Session on Integration of Statistics and Data Science (ISDS)
Chairs:
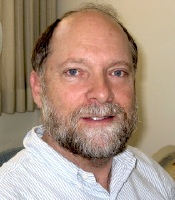 |
|
Roger Hoerl |
|
Union College, Schenectady, NY, USA |
|
Aims and Scope
Statisticians and those from machine learning or data science backgrounds often
approach modeling very differently. Statistical approaches generally begin with a
formal probability model, including an assumed density function for the error term.
Machine learning approaches are more often built upon numerical algorithms that
seem likely to fit the data in hand well. Unfortunately, this diversity in approach can
at times lead to an unhealthy “competition” between the disciplines, with each
promoting its approach as being universally superior. However, is it possible that
both approaches have merit, and that being familiar and skilled in both would lead
one to produce better models and better solutions? The purpose of this session is to
pursue such a hypothesis, and discuss how statistics and data science can both learn
from each other to enhance the field of analytics.
Topics of Interest:
- Expository papers on enhancement of analytics through more holistic approaches
- Case studies illustrating the integration of statistical and data science approaches
- Hybrid methodologies combining probability and algorithm based modeling
- Papers discussing how to structure the modeling approach based on the specifics of the problem at hand, i.e., explaining when particular methods are likely to work well