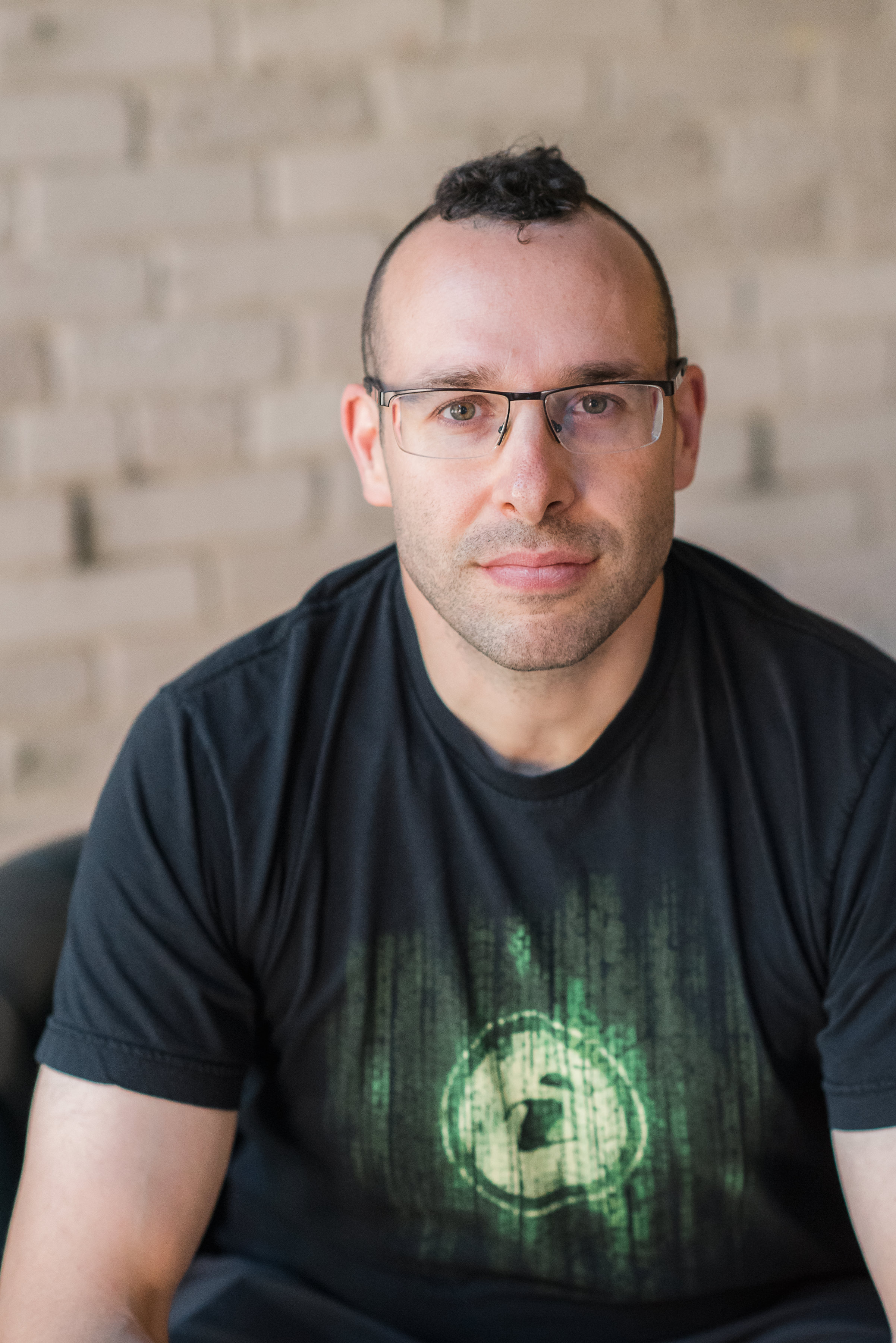
Adam White
My Students
If you are interested in joining my group as an MSc student, please apply directly to the MSc program. Do not contact me! I have no control over the admissions process: admission is based on grades, previous research experience, your research statement, and the quality of your reference letters. All students accepted to our MSc program get guaranteed TA funding. If you would like to work with me, then first apply to the MSc program, then contact once your are admitted and mention my favorite TV show Stargate.
Jacob Adkins (MSc)
Armin Ashrafi (MSc)
Oliver Diamond (MSc)
Tom Ferguson (Pdf)
Cameron Jen (MSc)
Scott Jordan (Pdf)
Ty Lazar (MSc)
Golnaz Mesbahi (MSc)
Samuel Neumann (PhD)
Parham Mohammad Panahi (Msc)
Kevin Roice (MSc)
Sam Scholnick-Hughes (MSc)
Han Wang (PhD)
Summer Students
Steven Tang (NSERC USRA)
Eric Xiong
Allumni
Jordan Coblin (MSc, 2024)
Banafshe Rafiee (PhD, 2024)
Matthew Schlegel (PhD, 2023)
Eugene Chen (MSc, 2023)
Subhojeet Pramanik (MSc, 2023)
Edan Meyer (MSc, 2023)
David Tao (MSc, 2022)
Samuel Neumann (MSc, 2022)
Derek Li (MSc, 2022)
Paul Liu (MSc, 2022)
Sina Ghiassian (PhD, 2022)
Raksha Kumaraswamy (PhD, 2021)
Matt McLeod (MSc, 2021)
Archit Sakhadeo (MSc, 2021)
Xutong Zhao (MSc, 2021)
Cam Linke (MSc, 2020)
Han Wang (MSc, 2020)
Niko Yasui (MSc, 2020)
Andrew Jacobsen (MSc, 2019)
Banafsheh Rafiee (MSc,2018)
Teaching
INT-D 161: AI Everywhere - Winter 2023
CMPUT 655: Reinforcement Learning I - Fall 2022
CMPUT 365: Introduction to Reinforcement Learning I - Fall 2021
CMPUT 607: Empirical Reinforcement Learning - Winter 2021
CMPUT 397: Reinforcement Learning I - Fall 2019
CMPUT 366: Intelligent Systems - Fall 2018
CMPUT 366: Intelligent Systems - Fall 2017
CMPUT 609: Reinforcement Learning - Fall 2017
CSCI-B 659: Reinforcement learning for Artificial Intelligence - Spring 2017
CSCI-B 659: Reinforcement learning for Artificial Intelligence - Spring 2016
Research
Keywords:
Continual Learning, Reinforcement Learning, Robotics, Knowledge Representation and
Intrinsic Motivation
Adam's research is focused on understanding the fundamental principles of learning in young humans, animals, and artificial agents in both simulated worlds and real industrial control applications. Adam's group is deeply passionate about good empirical practices and new methodologies to help determine if our algorithms are ready for deployment in the real world.
Curriculum vitae
My current CV can be found
here.
Journal Papers
Lo, C., Roice, K., Panahi, P. M., Jordan, S., White, A., Mihucz, G., Aminmansour, F., White, M. (2024) Goal-Space Planning with Subgoal Models, Journal of Machine Learning Research.
Wang, H., Miahi, E., White, M., Machado, M. C., Abbas, Z., Kumaraswamy, R., Liu, V., & White, A. (2024). Investigating the Properties of Neural Network Representations in Reinforcement Learning. AI Journal.
Sutton, R. S., Machado, M. C., Holland, G. Z., Timbers, D. S. F., Tanner, B., & White, A. (2023). Reward-respecting subtasks for model-based reinforcement learning. AI Journal
Ferguson, T. D., Fyshe, A., White, A., Krigolson, O. E. (2023). Humans adopt different exploration strategies depending on the environment. Computational Brain & Behavior
Janjua, M. K., Shah, H., White, M., Miahi, E., Machado, M. C., & White, A. (2023). GVFs in the Real World: Making Predictions Online for Water Treatment. Special issue on reinforcement learning for real life. Machine Learning
Tao, R. Y., Machado, M. C., White, A. (2023). Agent-State Construction with Auxiliary Inputs. Transactions on Machine Learning Research
Schlegel, M., Tkachuk, V., White, A. White, M. (2022). Investigating Action Encodings in Recurrent Neural Networks in Reinforcement Learning. Transactions on Machine Learning Research.
Wang, A., Sakhadeo, A., White, A., Bell, J. M., Liu, V., Zhao, X., Kozuno, T., Fyshe, A., White, A. (2022). No More Pesky Hyperparameters: Offline Hyperparameter Tuning for RL. Transactions on Machine Learning Research.
Patterson, A., White, A., White, M. (2022). A Generalized Projected Bellman Error for Off-policy Value Estimation in Reinforcement Learning. Journal of Machine Learning Research.
Rafiee, B., Abbas, Z., Ghiassian, S., Kumaraswamy, R., Sutton, R. S., Ludvig, E. & White, A. (2022). From eye-blinks to state construction: diagnostic benchmarks for online representation learning. Adaptive Behavior.
Schlegel, M., Jacobsen, A., Zaheer, M., Patterson, A., White, A., & White, M. (2021). General value function networks. Journal of Artificial Intelligence Research.
Linke, C., Ady, N. M., White, M., Degris, T., & White, A. (2020). Adapting behaviour via intrinsic reward: A survey and empirical study. Journal of Artificial Intelligence Research.
Modayil, J., White, A., Sutton, R. S. (2014). Multi-timescale Nexting
in a Reinforcement Learning Robot. Adaptive Behavior, 22(2):146--160.
Whiteson, S., Tanner, B., & White, A. (2010). The reinforcement
learning competitions. AI Magazine, 31(2): 81--94.
Tanner, B., & White, A. (2009). RL-Glue: Language-independent software for reinforcement-learning experiments. The Journal of Machine Learning Research, 10: 2133--2136.
Conference Papers
Edan Jacob Meyer, Adam White, and Marlos C. Machado (2024). Harnessing Discrete Representations for Continual Reinforcement Learning. The Reinforcement Learning Conference.
Scott M. Jordan, Samuel Neumann, James E. Kostas, Adam White, and Philip S. Thomas (2024). The Cliff of Overcommitment with Policy Gradient Step Sizes. The Reinforcement Learning Conference.
Parham Mohammad Panahi, Andrew Patterson, Martha White, and Adam White (2024). Investigating the Interplay of Prioritized Replay and Generalization. The Reinforcement Learning Conference.
Andrew Patterson, Samuel Neumann, Raksha Kumaraswamy, Martha White, and Adam White (2024). The Cross-environment Hyperparameter Setting Benchmark for Reinforcement Learning. The Reinforcement Learning Conference.
Jordan, S. M, White, A., Castro da Silva, B., White, M., Thomas, P. S. (2024). Position: Benchmarking is Limited in Reinforcement Learning Research.
International Conference on Machine Learning.
Rolnick, D., Aspuru-Guzik, A., Beery, S., Dilkina, B., Donti, P. L., Ghassemi, M., Kerner, H., Monteleoni, C., Rolf, E., Tambe, M., White. A. (2024) Position: Application-Driven Innovation in Machine Learning.
International Conference on Machine Learning.
Eugene Chen, Adam White, Nathan R. Sturtevant. (2023). Entropy as a measure of puzzle difficulty. Artificial Intelligence and Interactive Digital Entertainment.
Zaheer Abbas, Rosie Zhao, Joseph Modayil, Adam White, Marlos C. Machado. (2023) Loss of Plasticity in Continual Deep Reinforcement Learning. International Conference on Lifelong Learning Agents.
Vincent Liu, Han Wang, Ruo Yu Tao, Khurram Javed, Adam White, Martha White. (2023) Measuring and Mitigating Interference in Reinforcement Learning. International Conference on Lifelong Learning Agents.
Banafsheh Rafiee, Sina Ghiassian, Jun Jin, Richard S. Sutton, Jun Luo, Adam White. (2023) Auxiliary task discovery through generate-and-test. International Conference on Lifelong Learning Agents.
Chenjun Xiao, Han Wang, Yangchen Pan, Adam White, Martha White. (2023). The In-Sample Softmax for Offline Reinforcement Learning. International Conference on Learning Representations.
Samuel Neumann, Sungsu Lim, Ajin George Joseph, Yangchen Pan, Adam White, Martha White. (2023). Greedy Actor-Critic: A New Conditional Cross-Entropy Method for Policy Improvement. International Conference on Learning Representations.
Jiang, R., Zhang, S., Chelu, V., White, A., & van Hasselt, H. (2022). Learning Expected Emphatic Traces for Deep RL. AAAI Conference on Artificial Intelligence.
McLeod, M., Lo, C., Schlegel, M., Jacobsen, A., Kumaraswamy, R., White, M., & White, A. (2021). Continual auxiliary task learning. Advances in Neural Information Processing Systems.
Jiang, R., Zahavy, T., Xu, Z., White, A., Hessel, M., Blundell, C., van Hasselt, H. (2021). Emphatic Algorithms for Deep Reinforcement Learning. International Conference on Machine Learning.
Ghiassian S., Patterson A., Garg S., Gupta D., White A., White M.(2020). Gradient Temporal-Difference Learning with Regularized Corrections. International Conference on Machine Learning (ICML).
Ghiassian S., Rafiee B., Long Lo Y., White A. (2020). Improving Performance in Reinforcement Learning by Breaking Generalization in Neural Networks. International Conference on Autonomous Agents and Multi-Agent Systems (AAMAS).
Nath S, Liu V., Chan A., White A., White M. (2020). Training Recurrent Neural Networks Online by Learning Explicit State Variables. International Conference on Learning Representations (ICLR).
Wan Y., Zaheer M., Sutton R., White A., White M. (2019). Planning with Expectation Models. The International Joint Conference on
Artificial Intelligence (IJCAI).
Rafiee B., Ghiassian S., White, A., Sutton R. (2019). Prediction in Intelligence: An Empirical Comparison of Off-policy Algorithms on Robots
. The 18th International Conference on Autonomous Agents and Multiagent Systems (AAMAS).
Jacobsen A., Schlegel M., Linke C., Degris T., White, A., White M. (2019). Meta-descent for online, continual prediction
. AAAI Conference on Artificial Intelligence.
Kumaraswamy R., Schlegel M., White, A., White M. (2018). Context-dependent upper-confidence bounds for directed exploration
. Advances in Neural Information Processing Systems (NIPS).
Sherstan C., Bennett B., Young K., Ashley D., White, A., White M., Sutton R. (2018). Directly Estimating the Variance of the $\lambda$-Return Using Temporal-Difference Methods
. Conference on Uncertainty in Artificial Intelligence (UAI).
Pan Y., Zaheer M., White, A., Patterson A., White M. (2018). Organizing experience: a deeper look at replay mechanisms for sample-based planning in continuous state domains
. International Joint Conference on Artificial Intelligence (IJCAI).
Pan Y., White, A., White M. (2017). Accelerated Gradient Temporal Difference Learning
. AAAI Conference on Artificial Intelligence (AAAI).
Sherstan, C., Machado, M., ,White, A., Patrick P. (2016). Introspective Agents: Confidence Measures for General Value
Functions, Artificial General Intelligence (AGI).
White, A., White M. (2016). Investigating practical linear temporal difference learning. In International Conference on Autonomous Agents and MultiAgent Systems (AAMAS). [ CODE ]
White, M., White A. (2016) Adapting the trace parameter in reinforcement learning, In International Conference on Autonomous Agents and MultiAgent Systems (AAMAS).
White, A., Modayil, J., & Sutton, R. S. (2012). Scaling
life-long off-policy learning. In the IEEE International Conference on Development and Learning and
Epigenetic Robotics, 1--6.
[paper of distinction award]
Modayil, J., White, A., Pilarski, P. M., & Sutton, R. S. (2012). Acquiring a broad
range of empirical knowledge in real time by temporal-difference
learning. In the IEEE International Conference on Systems,
Man, and Cybernetics, 1903--1910.
Modayil, J., White, A., Sutton, R. S. (2012). Multi-timescale Nexting
in a Reinforcement Learning Robot. Presented at the 2012 International
Conference on Adaptive Behaviour, Odense, Denmark. To appear in: SAB
12, LNAI 7426, pp. 299-309, T. Ziemke, C. Balkenius, and J. Hallam,
Eds., Springer Heidelberg.
Sutton, R. S., Modayil, J., Delp, M., Degris, T., Pilarski, P. M.,
White, A., & Precup, D. (2011). Horde: A
scalable real-time architecture for learning knowledge from
unsupervised sensorimotor interaction. In The 10th
International Conference on Autonomous Agents and Multiagent
Systems: 2, 761--768.
White, M., & White, A. (2010). Interval
estimation for reinforcement-learning algorithms in continuous-state
domains. In Advances in Neural Information Processing Systems, 2433--2441.
Sturtevant, N. R., & White, A. M. (2007). Feature
construction for reinforcement learning in hearts. In
Computers and Games . Springer Berlin Heidelberg, 122--134
Preprints
Ghiassian S., Patterson A., White M.,, Sutton R. S., White A. (2019) Online Off-policy Prediction.
Other published works
Yasui N., Lim S., Linke C., White A., White M. (2019). An Empirical and Conceptual Categorization of
Value-based Exploration Methods. ICML Exploration in Reinforcement Learning
Workshop.
Pan Y., White, A., White M. (2017). Accelerated Gradient Temporal Difference Learning
. European workshop on reinforcement learning (EWRL).
Schlegel M., White, A., White M. (2017). Stable predictive representations with general value functions for continual learning
. Continual Learning and Deep Networks workshop at the Neural Information Processing System Conference.
White, A., & Sutton, R. S. (2014). GQ (lambda) Quick Reference Guide.
White, A., Modayil, J., & Sutton, R. S. (2014). Surprise and
curiosity for big data robotics. In Workshops at the
Twenty-Eighth AAAI Conference on Artificial Intelligence.
Modayil, J., White, A., Pilarski, P. M., Sutton, R. S. (2012). Acquiring
Diverse Predictive Knowledge in Real Time by Temporal-difference
Learning. International Workshop on Evolutionary and
Reinforcement Learning for Autonomous Robot Systems, Montpellier, France.
[Best paper award]
Modayil, J., Pilarski, P., White, A., Degris, T., & Sutton,
R. (2010). Off-policy knowledge maintenance for robots. In Proceedings
of Robotics Science and Systems Workshop (Towards Closing the Loop:
Active Learning for Robotics) : 55.
Theses
White, A. (2015) Developing a predictive approach to knowledge. Doctoral thesis, University of Alberta.
White, A. (2006) A standard system for benchmarking in reinforcement
learning. Master's thesis, University of Alberta.
See my
google scholar page for a list of my
publications that Google knows about.
Contact info
Office: 307 Athabasca Hall
Mail:
Department of Computing Science
University of Alberta
Edmonton, Alberta
Canada
|